Effective data management is crucial for enterprises looking to gain insights and drive decision-making. Two important concepts that often come up in this context are data discovery and data cataloging. While both play pivotal roles in data management, they serve different purposes and are used in distinct ways. This blog will explore the differences between data discovery and data cataloging, their unique benefits, and how they complement each other in a comprehensive data strategy.
What is Data Discovery?
Definition
Data discovery is the process of identifying patterns, correlations, and insights within datasets. It involves exploring and analyzing data to uncover hidden trends and relationships that can inform business decisions. Data discovery is often facilitated by interactive tools and visualizations, allowing users to drill down into data and perform ad-hoc analyses.
Key Components
- Data Profiling: Understanding the structure, content, and quality of data.
- Data Visualization: Using charts, graphs, and other visual tools to represent data insights.
- Exploratory Data Analysis (EDA): Techniques for analyzing data sets to summarize their main characteristics.
- Self-Service Analytics: Empowering users to explore data without needing extensive technical expertise.
Benefits of Data Discovery
- Enhanced Insight Generation: Quickly uncover hidden patterns and relationships within data.
- User Empowerment: Enables business users to explore data independently, reducing reliance on IT.
- Faster Decision-Making: Real-time data exploration leads to quicker insights and actions.
What is a Data Catalog?
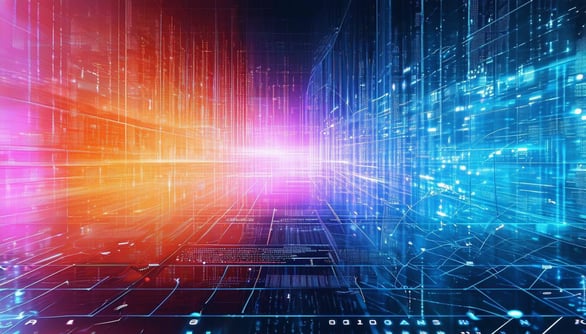
Definition
A data catalog is a comprehensive inventory of data assets within an organization. It provides metadata about data sets, including their source, usage, and lineage, making it easier for users to find and understand the data available to them. Data catalogs often include features like search functionality, data lineage tracking, and user annotations to facilitate data governance and collaboration.
Key Components
- Metadata Management: Collecting and organizing metadata about data assets.
- Data Lineage: Tracking the origin and transformations of data throughout its lifecycle.
- Search and Discovery: Enabling users to find relevant data quickly through search functionality.
- Data Governance: Ensuring data quality, compliance, and security through policies and controls.
Benefits of a Data Catalog
- Improved Data Governance: Provides a centralized repository for managing data policies and ensuring compliance.
- Enhanced Data Accessibility: Makes it easier for users to find and understand data assets within the organization.
- Collaboration and Sharing: Facilitates collaboration by allowing users to annotate and share data insights.
Data Discovery vs Data Catalog: Key Differences
Objective
Data Discovery
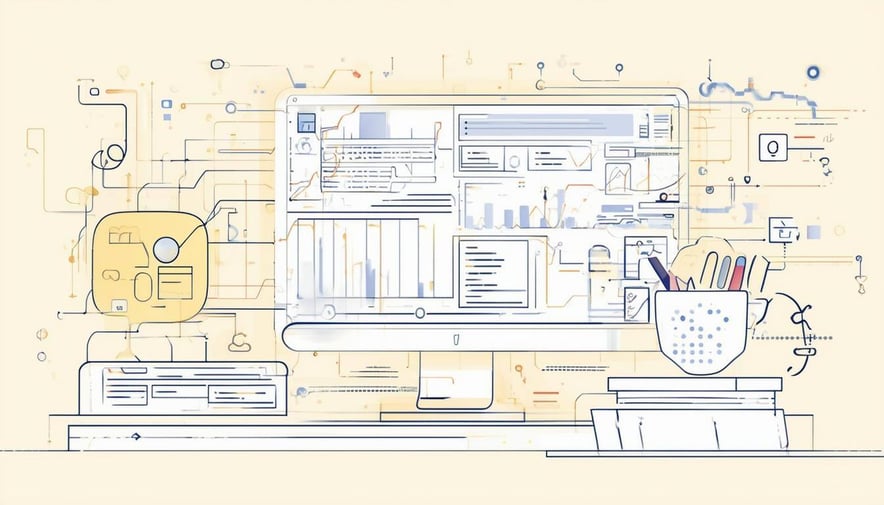
Exploratory Focus: The primary goal of data discovery is to explore and analyze data to uncover hidden patterns, trends, and insights. This process is inherently investigative and allows users to interact with data in a dynamic way. The focus is on finding relationships and anomalies that might not be apparent in pre-defined reports or traditional analysis methods.
- Uncover Hidden Insights: Through data discovery, users can identify unexpected correlations and trends that can inform strategic decisions.
- Flexible Exploration: Users can navigate through data freely, asking new questions and pursuing lines of inquiry as they arise, without being constrained by predefined structures.
User-Driven Analysis: Data discovery emphasizes a user-driven approach where business users, analysts, and non-technical staff can independently explore and analyze data. This autonomy reduces the dependency on IT and allows users to derive insights quickly, responding to business needs in real-time.
- Empowers Users: By providing tools that are accessible to non-technical users, data discovery empowers a broader range of employees to participate in data analysis.
- Rapid Insights: The ability to perform ad-hoc analysis and create custom visualizations enables users to generate insights swiftly, facilitating timely decision-making.
Data Catalog
Organizational Focus: The main objective of a data catalog is to organize and inventory all data assets within an organization. It aims to create a comprehensive and easily searchable repository that provides users with a clear understanding of what data is available and how it can be used.
- Centralized Data Repository: A data catalog serves as a single source of truth, consolidating information about data assets from various sources.
- Improved Accessibility: By cataloging data assets, it becomes easier for users to find and access the data they need, enhancing overall productivity.
Metadata Management: Data catalogs focus on managing metadata—the data about data. This includes details such as data source, creation date, usage, and lineage. Effective metadata management improves data governance and ensures that data assets are properly documented and understood.
- Contextual Information: Metadata provides essential context that helps users understand the provenance, structure, and relevance of data.
- Data Governance: Managing metadata helps ensure compliance with data governance policies and standards, maintaining data quality and security.
Approach
Data Discovery
Interactive and Visual: Data discovery relies heavily on interactive and visual tools for analysis. These tools, such as dashboards, charts, and graphs, allow users to visualize data trends and patterns easily. The visual nature of these tools makes data more accessible and comprehensible to non-technical users.
- Engaging Visuals: Interactive visualizations help users to see data in new ways, making it easier to identify trends and outliers.
- User-Friendly Interfaces: Tools designed for data discovery often feature intuitive interfaces that simplify the exploration process.
Ad-Hoc Analysis: Supports the ability to perform ad-hoc queries and analyses, enabling users to ask spontaneous questions and explore data in real-time. This approach is crucial for addressing immediate business questions and hypotheses without waiting for formal reports to be generated.
- Immediate Inquiry: Users can investigate specific questions as they arise, leading to quicker problem-solving and insight generation.
- Dynamic Analysis: The flexibility to adjust queries and explore different data dimensions on the fly fosters a deeper understanding of the data.
Data Catalog
Systematic and Structured: Data cataloging involves a systematic and structured approach to collecting and organizing metadata. This ensures consistency and reliability in how data assets are documented and managed.
- Standardized Metadata: Consistent metadata formats and structures help maintain clarity and accuracy across the data catalog.
- Organized Documentation: Systematic organization makes it easier to maintain and update the catalog as new data assets are added.
Search and Retrieval: Provides robust search functionality to quickly locate data assets. This search capability is essential for users to efficiently find the data they need for their analyses and decision-making processes.
- Efficient Search: Advanced search features allow users to find relevant data quickly using keywords, tags, and filters.
- Comprehensive Retrieval: Users can access detailed information about data assets, including metadata and lineage, to better understand the data’s context and usage.
Data Types
Data Discovery
Structured and Unstructured Data: Data discovery tools are designed to handle a variety of data types, including structured data from databases and unstructured data such as text, images, and social media content. This versatility enables a more holistic analysis of different data sources.
- Diverse Data Sources: Capable of integrating data from multiple sources, enhancing the breadth and depth of analysis.
- Advanced Algorithms: Uses machine learning and other advanced techniques to process and analyze unstructured data, identifying patterns and insights that might be missed by traditional methods.
Pattern Recognition: Employs sophisticated algorithms to identify patterns and trends within diverse datasets. This capability is crucial for uncovering insights that can drive strategic decisions.
- Machine Learning: Utilizes machine learning models to detect complex patterns and correlations.
- Data Mining: Engages in data mining practices to extract useful information from large datasets.
Data Catalog
Primarily Structured Data: Focuses on cataloging structured data assets, though it can also include metadata about unstructured data. The primary aim is to provide a clear and comprehensive inventory of an organization’s structured data resources.
- Structured Data Focus: Emphasizes well-defined data formats typical of relational databases and data warehouses.
- Metadata for Unstructured Data: Can include metadata for unstructured data, providing context and information about these assets even if they are not directly cataloged.
Metadata Focus: Centers around managing metadata, providing detailed information about data sources, usage, and transformations. This focus helps users understand the data’s lineage and governance aspects.
- Comprehensive Metadata: Includes extensive details about data attributes, sources, and history.
- Contextual Insights: Metadata offers insights into the data’s context, making it easier for users to assess its relevance and reliability.
Users
Data Discovery
Business Users and Analysts: Designed for use by business users, data analysts, and non-technical staff. The intuitive and user-friendly tools enable these users to explore and analyze data independently, without needing deep technical knowledge.
- Broad User Base: Accessible to a wide range of users within the organization, promoting widespread data-driven decision-making.
- Empowerment: Empowers users to perform their own analyses, reducing the bottleneck often created by reliance on IT departments.
Empowerment and Independence: Encourages users to conduct their own data explorations and analyses, fostering a culture of self-service analytics. This independence accelerates the pace of insights and reduces the burden on IT resources.
- Self-Service Analytics: Tools designed for self-service enable users to generate their own reports and visualizations.
- Rapid Insights: Users can quickly derive insights from data, facilitating more agile decision-making processes.
Data Catalog
Data Stewards and IT Professionals: Typically used by data stewards, IT professionals, and data governance teams to manage and oversee data assets. These users are responsible for maintaining data quality, security, and compliance.
- Specialized Roles: Involves users with specialized knowledge of data management and governance practices.
- Governance Focus: Ensures that data assets are properly documented, secured, and compliant with regulatory standards.
Collaboration and Governance: Facilitates collaboration among various stakeholders and ensures robust data governance through organized metadata and comprehensive documentation.
- Collaboration Tools: Features that allow users to annotate, comment on, and share data insights foster collaboration.
- Governance Mechanisms: Provides tools and frameworks to enforce data governance policies and standards across the organization.
How Data Discovery and Data Catalogs Complement Each Other
While data discovery and data cataloging serve different purposes, they are complementary processes that together enhance an organization’s data management capabilities.
Synergistic Benefits
- Enhanced Data Accessibility: Data catalogs make it easy to find and understand data, providing a foundation for effective data discovery.
- Improved Data Quality and Governance: By cataloging data and managing metadata, organizations ensure that data used in discovery is accurate and compliant with governance policies.
- Comprehensive Data Strategy: Combining data discovery and cataloging allows organizations to leverage the strengths of both, driving better insights and more informed decision-making.
Implementation Strategies
- Integrated Platforms: Use integrated data management platforms that support both data discovery and cataloging functionalities.
- User Training and Adoption: Ensure that users are trained in both data discovery tools and data cataloging practices to maximize the benefits of both.
- Data Governance Framework: Implement a robust data governance framework that incorporates both discovery and cataloging to ensure data quality, security, and compliance.
Understanding the differences between data discovery and data cataloging is crucial for effective data management. Data discovery focuses on exploring data to uncover hidden insights, while data cataloging organizes and inventories data assets to improve accessibility and governance. By integrating both approaches, enterprises can enhance their data strategy, driving better insights, compliance, and decision-making.
Key Takeaways
- Data Discovery: Focuses on exploratory analysis to find hidden patterns and trends using interactive and visual tools.
- Data Catalog: Organizes and inventories data assets, providing metadata and ensuring data governance.
- Complementary Roles: Together, data discovery and cataloging enhance data accessibility, quality, and governance.
- Implementation: Use integrated platforms, provide user training, and implement a strong data governance framework.
- Strategic Value: Leveraging both approaches drives better insights and more informed decision-making.
About Shinydocs
Shinydocs automates the process of finding, identifying, and actioning the exponentially growing amount of unstructured data, content, and files stored across your business.
Our solutions and experienced team work together to give organizations an enhanced understanding of their content to drive key business decisions, reduce the risk of unmanaged sensitive information, and improve the efficiency of business processes.
We believe that there’s a better, more intuitive way for businesses to manage their data. Request a meeting today to improve your data management, compliance, and governance.
